CHING'S MARKOV CHAIN MODELS
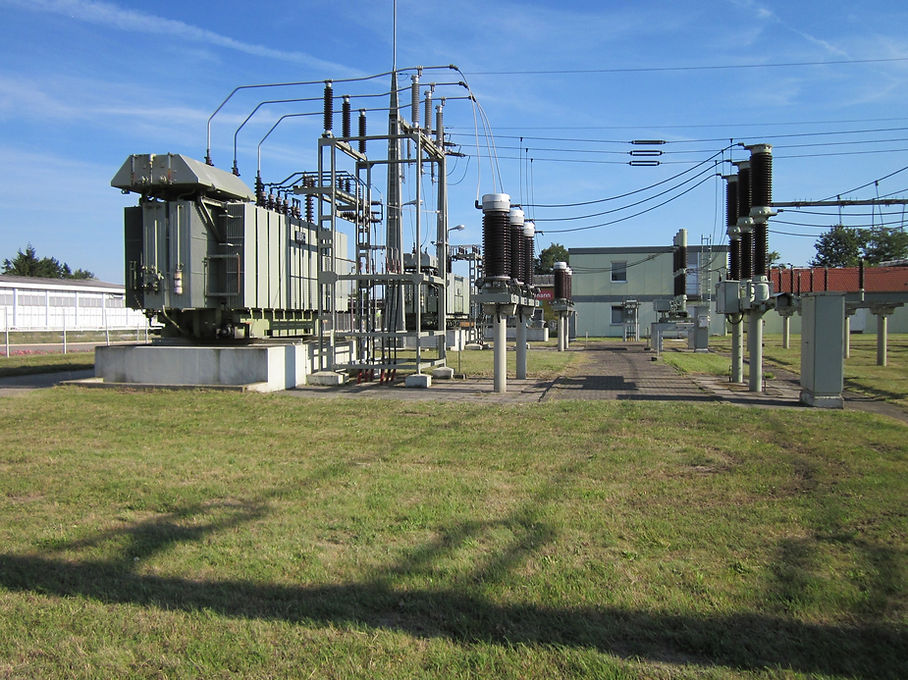
ELECTRICITY FORECASTING
W. Zhao, J. Wang and H. Lu (2014). Combining Forecasts of Electricity Consumption in China with Time-varying Weights Updated by a High-order Markov Chain Model. OMEGA, 45: 80-91.
https://www.sciencedirect.com/science/article/pii/S0305048314000036
In Zhao et al. (2014), the authors considered the problem of forecasting electricity consumption in China. They proposed to use a combining method which makes good use of different forecasting models, this gives a better prediction of electricity consumption in China. At the same time, due to the instability problem, fixed weights may perform poorly. So, the combining weights are suggested to be changed according to the time-varying underlying process. In order to obtain the combining weights, they predict them based on the categorical sequences of combining weights in the sample period. For modeling this type of sequence, it has been shown that the high-order Markov chain model is a promising approach. Meanwhile, compared with a conventional model for nth order Markov chain which requires (m-1)mⁿ parameters, the parsimonious high-order Markov chain model in Ching et al. (2004, 2008), just requires n+nm² parameters. Furthermore, the model is generalized by allowing the transition matrix Q to vary with different lags to have a better prediction power. With these two advantages, the parsimonious high-order Markov chain model and estimation method are employed in Zhao et al. (2014) to predict the out-of-sample combining weights through extrapolating the in-sample weights.
The Markov chain is the combining weights of different individual models varying with time. The original categorical data sequence is a sequence of in-sample combining weights by solving one optimization problem for each time point. The combining weight vector at each time point is treated as a state probability distribution. The parsimonious high-order Markov chain model along with the estimating methods of the i-th step transition probability matrix Qi and coefficient i are followed to obtain the combining weight vector.
The performance of the parsimonious high-order Markov chain based time-varying weighted average model (HM-TWA) model in Zhao et al. (2014) for forecasting electricity in China has been compared with eight models, including three common methods (back-propagation network (BPN), least squares support vector regression (LSSVR) and seasonal autoregressive integrated moving average SARIMA)), three individual models (predetermined seasonal term method (PSTM), predetermined trend term method (PTTM) and Holt-Winters method (HWM)), two traditional combining models (simple average (SA) and weighted average (WA)), and one existing time-varying combining method, namely time-varying sum of squared error (TSSE) method. According to the three criteria, root mean square error (RMSE), mean absolute error (MAE) and mean absolute percentage error (MAPE), with the predetermined parameters for BPN, LSSVR and SARIMA, HM-TWA has different degrees of improvement when compared with other models for both one-month-ahead and one-year-ahead forecasting. In particular, the one-step-ahead forecasting results during the period from June 2011 to May 2012 show that there are noticeable improvements for HM-TWA when compared with BPN, LSSVR, SARIMA, PSTM, PTTM, HWM, SA, WA and TSSE, and the MAPE have been reduced by 76.98%, 68.87%, 18.77%, 71.24%, 23.01%, 25.91%, 28.47%, 24.55% and 16.62%, respectively.
W. Ching, E. Fung and M. Ng (2004). Higher-order Markov Chain Models for Categorical Data Sequences, Naval Research Logistics, 51: 557-574.
https://doi.org/10.1002/nav.20017
W. Ching, M. Ng and E. Fung (2008). Higher-order Multivariate Markov Chains and
Their Applications, Linear Algebra and Its Applications, 428(2–3):492-507.
https://www.sciencedirect.com/science/article/pii/S0024379507002169