CHING'S MARKOV CHAIN MODELS
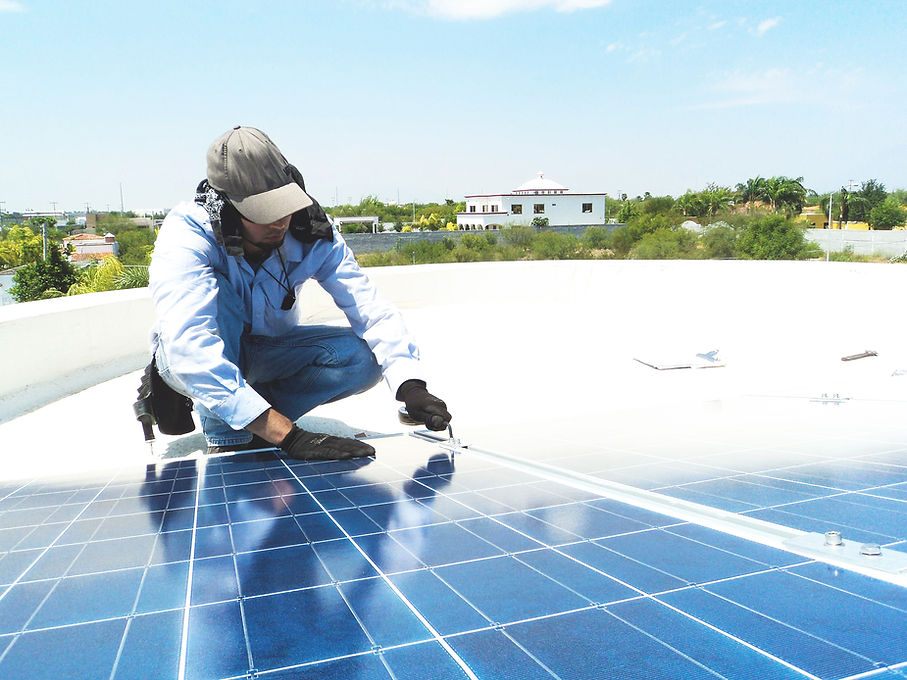
PV POWER GENERATION FORECASTING
M. J. Sanjari and H. Gooi (2017). Probabilistic forecast of PV power generation based on higher order markov chain. IEEE Trans. Power Systems, 32: 2942–2952.
https://ieeexplore.ieee.org/document/7592462
In Sanjari et al. (2017), the authors considered the problem of forecasting the probability distribution function (PDF) of the generated power of Photovoltaic (PV) systems. They presented a method based on the higher-order Markov chain (HMC) model. In each operating point, an HMC is developed based on the categorized historical data of PV power. The 15-min ahead PDF of the PV output power is forecasted through the Gaussian mixture method (GMM) by combining several distribution functions and by using the coefficients defined based on parameters of the HMC-based model.
In the HMC model, the categorical data sequence is the data sequence of PV output power. Since the output datum are continuous, so they are discretized beforehand. The parsimonious higher-order multivariate Markov chain model along with the parameter estimation method in Ching et al. (2004, 2008) is then implemented to model the probabilistic relationship of PV generated power in subsequent time intervals. With the help of the parsimonious higher-order multivariate Markov chain model along with the parameter estimation method in Ching et al (2004, 2008), the required parameters are significantly less than the conventional one. Also, the parameter estimation problem can be reduced to linear programming problem which is easier to handle.
In Sanjari et al. (2017), the authors evaluated their model based on the PV generated power, solar radiation and ambient temperature data collected at St. Lucia Campus, University of Queensland, Australia. The values of the mentioned variables recorded for the years 2014 and 2015 are adopted. The former is used to train the forecast model and the latter is used to test the performance of the proposed method. The authors tested the performance of the method in two scenarios. In scenario 1, the forecast model is built based on the complete training set of the data, i.e., data of PV output power in the year 2014 and is used to forecast the PV power PDF in the year 2015. The fitted distributions over the data measured in two months are displayed in Fig. 1. The proposed method provides a better fit for the histograms of the PV output as compared to other distributions. To show the capability of the proposed model to adaptively update the model parameters, in scenario 2 the parameters of the developed model in scenario 1 are updated at the end of every hour of the year 2015 by using the measured PV power at that time interval. Fig. 2 compares the performance of different forecasting methods over data of the year 2015. It is observed that the proposed method outperforms the other models at different forecasting horizons. With these promising results, the authors in Sanjari et al. (2017) concluded that the proposed method can estimate PDF of the PV generated power accurately irrespective of the PV system operating point. Moreover, the model parameters can be updated over time leading to the higher accuracy in PDF forecast of PV power.
Fig. 1
Taken from “Probability distribution forecast of the PV generated power at different months of the year 2015 using different fittings/approaches.” M. J. Sanjari and H. Gooi (2017). Probabilistic forecast of PV power generation based on higher order Markov chain (2017) https://ieeexplore.ieee.org/document/7592462
Fig.2
Taken from “Quantile-quantile plot of the reliability diagram related to the PV power distribution forecast.” M. J. Sanjari and H. Gooi (2017). Probabilistic forecast of PV power generation based on higher order Markov chain (2017) https://ieeexplore.ieee.org/document/7592462
W.Ching, E. Fung, and M. Ng (2004) Building higher-order Markov chain models with EXCEL, Int. J. Math. Educ. Sci. Technol:35 921–932
W. Ching, E. Fung, and M. Ng (2008) Higher-order Multivariate Markov Chains and Their Applications, Linear Algebra and its Applications, 15, 492-507.
https://www.sciencedirect.com/science/article/pii/S0024379507002169

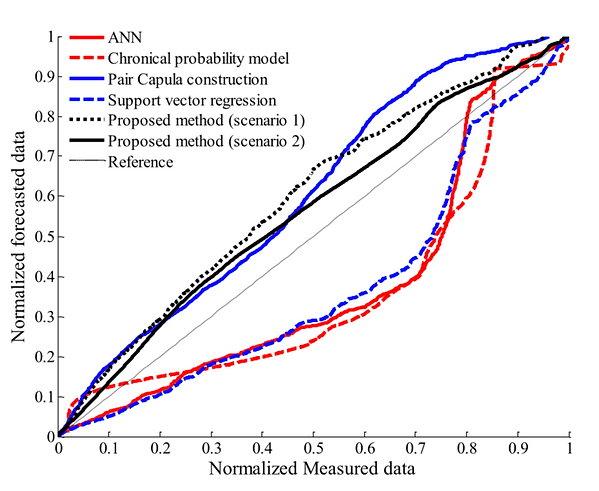